A small island in the state of Washington houses a group of unlikely residents: they are all men the state considers its most dangerous sex offenders.
McNeil Island, nestled in Puget Sound, is unpopulated except for the 214 people who live at the special commitment center, a facility for former prison inmates. All men have served their sentence and yet, due to a controversial legal mandate, they remain confined indefinitely.
The only way on and off the small island is a passenger-only ferry, which makes the 15-minute trip every two hours. The ferry docks at a defunct prison on the island and a bus takes employees and visitors to the facility a few miles inland. Along the way, the bus passes an overgrown baseball field and boarded-up houses, remnants of the prison employees and their families who called the island home until the prison closed in 2011.
Few people who live in the region know about the island and its unusual residents, and even fewer know about the equally unusual law that put them there.
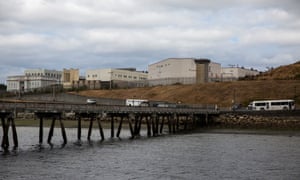
Kelly Canary, an attorney who represents some of the men confined to the commitment center, said people are often shocked when they discover that “even after [offenders have] served their time and get out of prison, they can be civilly committed and detained for the rest of their life.”
Each of the residents has previously been convicted of at least one sex crime – including sexual assault, rape and child molestation. A court has then found them to meet the legal definition of a “sexually violent predator”, meaning they have a mental abnormality or personality disorder that makes them likely to engage in repeat sexual violence.
Civil commitment centers, which exist in fewer than half of US states, are meant as a community safeguard and a means of providing treatment for the offenders. But they’re riddled with controversies. Criminal justice reform advocates fear the implications of predicting future risk and basing confinement on what someone might do.
On top of that, these costly facilities also have low release numbers, making little known about whether they are doing anything to keep communities safer.
The people sent to the special commitment center on McNeil Island are called “residents”, not inmates, though it is difficult to distinguish the facility from a prison. Rows of barbed-wire fences pen the grounds and counselors check residents every hour to make sure they are adhering to the facility’s rules.
“In most ways, it’s worse because the illusion is it’s not prison,” Calvin Malone, one of the residents, told me.
During the 1970s and 1980s, Malone worked as a Boy Scout troop leader in various states across the country, as well as with an organization that works with at-risk youth. In these roles, he molested numerous boys and was convicted of sex crimes in California, Oregon and Washington.
When he entered prison, where he spent 20-plus years, he was addicted to heroin.
“I didn’t care about anything,” he said. “I put on a facade that I did, that’s how I had to navigate.”
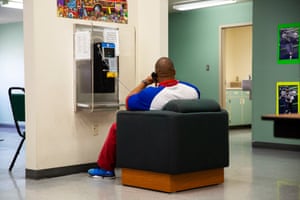
About a year in, he learned about Buddhism from a magazine. He started meditating and corresponding with Buddhists outside of prison. During his sentence he also underwent sex offender therapy and he said that the combination of Buddhist teachings and treatment helped him gain perspective on who he was and what he’d done.
“I convinced myself all these years that I was a great guy … I justified, I manipulated, I minimized. I did all the things an offender will do to justify behavior to myself,” he said. “[Treatment and meditation] raised my level of empathy to a point where I understood the impact of my offending behavior and the ultimate damage that was done.”
Malone said he doesn’t like talking about how he feels in terms of shame or guilt. Those emotions, he said, have more to do with how he feels about himself. Instead, he said he feels regret.
“To regret is to understand what you’ve done and the losses that have occurred because of your actions and it allows you the space to move forward so that you’re not wallowing,” he said. “I have a tremendous amount of regret.”
Washington’s civil commitment center is unique, not only for its banished-to-an-island affinity, but because it was the first of its kind.
On 26 September 1988, convicted sex offender Gene Raymond Kane abducted, raped and murdered 29-year-old Diane Ballasiotes. At the time of the incident, Kane had been released from prison to a work release center.
Ballasiotes’s death, followed by two other disturbing sexual assaults by different assailants, fueled a public outcry that eventually led the governor to sign the Community Protection Act of 1990. The act was a package of laws aimed at sex offenders, including tougher sentences, a sex offender registration and the creation of a procedure that allowed authorities to indefinitely lock up sex offenders when a court believes them a continued threat to the community.
Since then, 19 other states have enacted similar civil commitment laws. There are more than 5,200 people civilly committed in the US, according to a 2017 survey of 20 civil commitment centers.
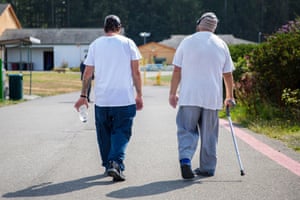
About half of the states with such laws allow the commitment of individuals who offended as juveniles. Many of those committed are diagnosed with a general paraphilia, a condition in which a person’s sexual arousal and gratification depends on behavior considered atypical or extreme.
Mental health professionals are split as to whether this diagnosis as a commitment standard is appropriate, Dr Shan Jumper, president of the Sex Offender Civil Commitment Programs Network (SOCCPN), told me. Many of the sexually violent predator evaluations for men convicted of rape are diagnosed with “paraphilia – not otherwise specified”, Jumper said. The controversy lies, he said, in the fact that the Diagnostic and Statistical Manual of Mental Disorders does not have a specific classification for adults who are sexually aroused by those who don’t consent.
Fundamentally, these laws are about predicting a person’s future risk, which comes with its own moral and philosophical dilemmas.
To do this, states use actuarial scales, which predict an offender’s risk in the same way that car insurance companies determine rates. A widely used tool, the Static-99R, produces a score based on a number of mostly unchangeable things including criminal and relationship history.
The results, along with other evidence such as expert testimony from psychologists, is presented to a judge or jury, who determine whether the offender meets the criteria.
But SOCCPN concedes that current research and actuarial tools are not designed to predict individual risk. “To some extent the criminal justice system is requiring opinions to be made, decisions to be made, that go somewhat beyond the knowledge base that we have,” Dr Michael Miner, a professor of human sexuality at the University of Minnesota and past president of the Association for the Treatment of Sexual Abusers (ATSA), told me.
Miner said that aside from the problems with risk assessment, he questions the entire civil commitment process.
“You either have a mental defect that makes it unlikely that you can control your behavior and therefore you’re not guilty by reason of insanity, or you’re responsible for your behavior,” he said.
“It seems to me that a more honest system would, at the front end, say: ‘we just think you’re a bad guy and we’re not going to let you out, we’re going to give you a life sentence.’ I’m not advocating for life sentences for sex offenders, but that seems like a more honest route.”
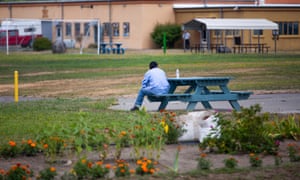
The US supreme court has upheld the constitutionality of civil commitment statutes three times. ATSA doesn’t take an official position for or against civil commitment centers.
Civil confinement in Washington cost $185,136 per resident in 2018. That is about five times more per person than the average cost to confine one Washington prisoner in 2017, the most recent data.
Miner points out that sex offenders have a relatively low reoffense rate. Of the offenders convicted of rape and sexual assault who were released from prison in 30 states in 2005, an estimated 5.6% were rearrested for rape or sexual assault five years later, according to a 2016 study by the US Department of Justice. The same statistics for other types of crimes were much higher. Fifty four percent of property offenders were rearrested for a property crime and 33% of drug offenders were rearrested for a drug crime.
“There is a moral panic around sexual crimes and [the public believes] that these people pose an extraordinarily high level of danger,” Miner said. “To the frustration of me and a lot of other people who are trying to come up with commonsense ways of preventing sexual violence, the message that most of these people are not really all that risky isn’t something that people seem to listen to.”
In addition to safety for the larger community, civil commitment centers are designed to provide sex offenders with treatment. This is usually based in cognitive behavioral therapy, which aims at challenging distorted thoughts and regulating emotions to change behavior.
In therapy at the SCC, offenders are encouraged to disclose all of their sexual deviance to help understand the scope of their problem. Clinicians then target the factors that make them vulnerable to reoffend. The ultimate goal isn’t to eliminate urges but to mitigate risk by modifying thoughts and emotions to change destructive behavior.
“We focus on what we can change,” said Dr Elena Lopez, chief of resident treatment at the SCC. “It’s different for every person. They might have their own internal personal hurdles that keep them from progressing, personality traits, motivation, acute medical conditions, stressors.”
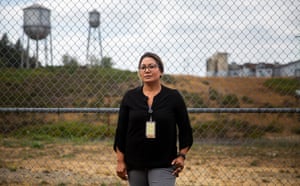
Though there is limited data on sex offender treatment, research shows that it is promising in terms of reducing recidivism.
All civil commitment centers offer treatment, but participation isn’t mandatory. On McNeil Island, about 62% of the residents participate in treatment.
Working with this population can be challenging but also rewarding, Lopez said, especially when taking into account the small incremental changes that happen over time.
“We as clinicians can’t expect quick change because they didn’t get here overnight. We’re talking about long histories of engaging in this type of behavior, this type of interaction, maybe even this style of seeing the world that makes it hard to keep others and themselves safe,” she said. “I take great pride in knowing that I’m keeping the community safe, but I’m also helping people shift and change their lives to be meaningful and safe.”
Once someone is labeled a sexually violent predator and committed to a civil commitment center, it can be difficult for them to get released.
In most states, a person who is civilly committed has the right to an annual review, in which a court goes over each offender’s history and treatment progress to consider release.
At the SCC, offenders have a yearly evaluation by a forensic team, which reviews documents, interviews offenders and clinicians and collects results from a polygraph and penile plethysmography, a tool designed to measure sexual arousal.
Dr Holly Coryell, chief of forensic services at the SCC, said forensic evaluators are ultimately looking to answer three psycho-legal questions: does the person continue to meet the legal criteria for a sexually violent predator? Are less restrictive alternatives in the person’s best interest? Can conditions be imposed that would adequately protect the community?
Forensic evaluators answer these questions in a recommendation that is then forwarded to the court, creating the opportunity for release hearings.
But arguing that a sex offender should be released to the community can be an uphill battle.
“The state gets to say, at the beginning of trial, he is a sexually violent predator,” Canary said. “Getting the jury to be on board with [the idea that he might not be any more] is pretty difficult.”
Though offenders are encouraged to disclose everything in treatment, they sign away their confidentiality and everything revealed to a clinician can be used as evidence. Much like an alcoholic is encouraged to admit they’re always in recovery, offenders are taught that treatment is ongoing and that consistent self-monitoring is key. Canary said that many of her clients readily admit the fact that they’re always a risk to the community, a line of thinking that helps them stay self-aware.
“But then the jury hears that,” Canary said. “You have your client saying, ‘Well, sure, I’m a risk to reoffend.’ It’s something that jurors just don’t like to hear … once they hear that from your client, it’s kind of hard to put that into perspective.”
Through the Washington court process, a civilly committed person can be released to less restrictive alternatives, which typically include outpatient treatment and tight restrictions, or they can be released without conditions.
The number of people released nationally from these facilities through either avenue is historically low. On average, these facilities house about 260 people. Of the 16 states that provided release numbers to a 2017 survey of civil commitment centers, the average number of people released from a facility per year was seven. Five states released an average of less than one person per year.
The low number of people released from these facilities makes it hard to research the effectiveness of these laws and these facilities.
As for Malone, he doesn’t participate in treatment. Now in his 60s, he said he has benefited from treatment in prison and prefers to focus on other things to make life on McNeil Island better. He has led a few lawsuits aimed at improvements, one dealing with tobacco use and another calling into question the facility’s water quality. He is heavily involved in the Buddhist community and spent years petitioning to have a pagoda built. He said he enjoys meditating among the gardens that surround the ornate structure.
“I’ve accepted the fact that this could be my last stop. I could die here,” he said. “The only thing I would love to do is have the opportunity to talk more about what my future would be rather than to constantly revisit something that occurred decades ago … It makes it difficult to do rehabilitation work on yourself when you’re still stuck in that experience.”